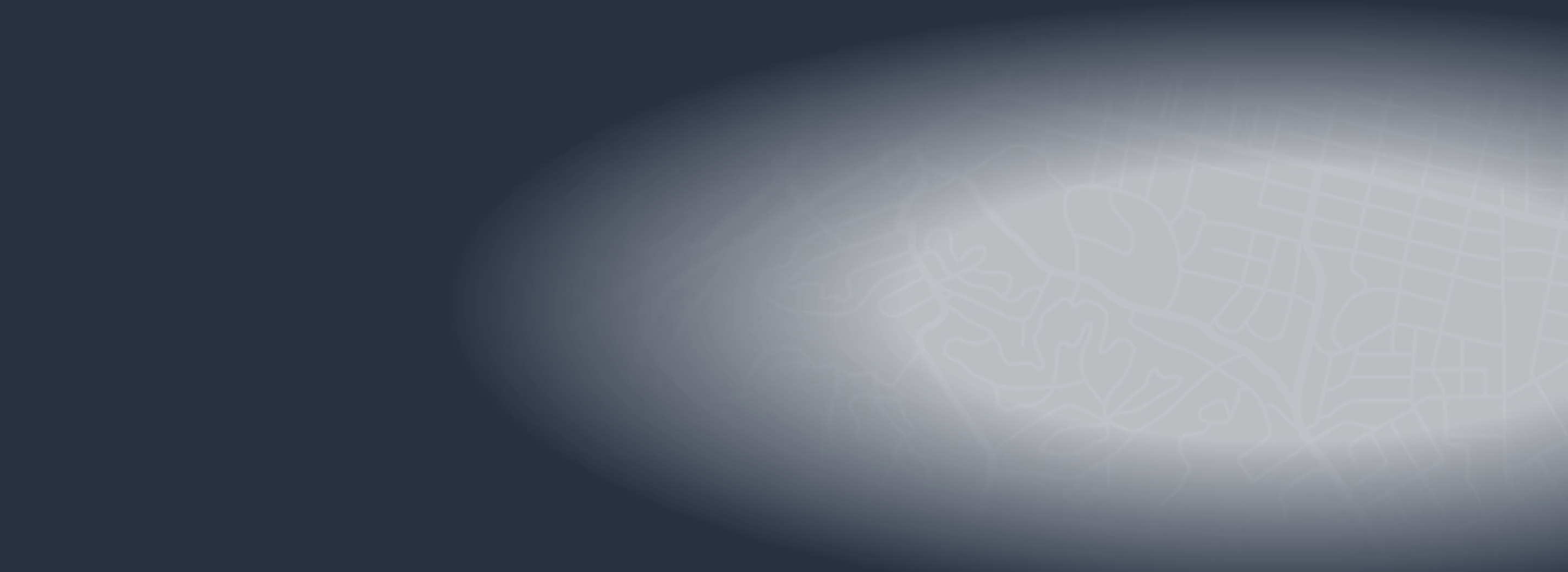
Traffic Calming
Optimizing School Zone Speed Camera Placement with Geospatial AI
Geospatial AI helps cities strategically place school zone speed cameras – reducing speeding and improving child safety
Geospatial AI helps cities strategically place school zone speed cameras using traffic, pedestrian, and crash data – reducing speeding and improving child safety.
School zone safety is a growing concern for cities and communities. As urban areas expand and traffic volumes rise, keeping children safe around schools is becoming increasingly complex. During morning drop-off and afternoon pick-up, roads near schools often see a perfect storm of risk factors: narrow sidewalks, hurried drivers, and unpredictable congestion. The data paints a troubling picture – according to the National Highway Traffic Safety Administration, 1 in 5 children under 15 killed in traffic crashes are pedestrians, many of them near schools.
Studies have found that 50–90% of motorists travel above the posted speed limit in school zones, despite the lowered limits. This is alarming because a pedestrian hit by a vehicle at 20 MPH has less than a 10% risk of fatal injury, but at 40 MPH that risk jumps to over 50%. Clearly, traditional measures are struggling to curb dangerous speeding where it matters most.
Communities have long relied on tools like flashing school zone signs, speed bumps, crossing guards, and the occasional police presence to slow drivers. While these traditional traffic calming solutions do play an important role, they are often reactive, static, and unable to adapt to real-time risks. A speed bump slows every car at a fixed location, but it can’t target the most problematic times of day. A crossing guard can help children cross one intersection, but cannot enforce drivers slowing down along an entire corridor. And manual enforcement by officers is resource-intensive and limited in coverage. Moreover, choosing where to place a speed camera or officer is typically based on past incidents or complaints, rather than a comprehensive view of risk factors. This conventional approach can leave gaps – speeding hotspots that go unchecked and times of day when risky behavior spikes without adequate enforcement.
The growing need for smarter school zone enforcement is evident. Cities are looking for ways to proactively prevent accidents, rather than only reacting after tragedy strikes. This is where geospatial artificial intelligence (AI) comes into play. By leveraging data and advanced analytics, public leaders and transportation professionals can pinpoint exactly where and when to enforce speed limits around schools for maximum impact. Instead of guesswork or one-size-fits-all measures, data-driven tools enable strategic placement of speed cameras to address the worst dangers first.
In the sections below, we’ll explore how Urban SDK’s geospatial AI platform helps cities analyze complex traffic patterns, identify high-risk zones, and determine the most effective locations for speed camera installations – ultimately reducing speeding and creating safer streets for children.
How Geospatial AI Optimizes Speed Camera Placement
Geospatial AI combines location-based data with artificial intelligence to analyze complex spatial patterns and make intelligent recommendations. In the context of school zone safety, Geospatial AI can sift through massive amounts of information – from traffic speeds to walking patterns – to highlight the ideal spots to install speed cameras. Instead of relying on intuition or isolated data points, city officials can use these AI-driven insights to target enforcement exactly where it will save the most lives and prevent the most accidents.
Here’s how Urban SDK’s geospatial AI platform analyzes multiple data streams to optimize camera placement:
Traffic Speeds and Patterns
Geospatial AI continuously aggregates speed data from connected vehicle sensors, GPS probes, and traffic counters on every road in a city. Instead of a one-time speed study, officials get a dynamic view of how fast cars are actually going near each school, hour by hour. This helps identify chronic speeding hotspots. For example, the AI might reveal that on a certain street adjacent to a school, average speeds are 10 mph over the limit during the 7-8 AM drop-off window every weekday. If 85% of drivers are exceeding the school zone limit at a specific location, that street becomes a prime candidate for a camera.
Urban SDK provides precise hourly speed data on every road, enabling agencies to pinpoint where and when speeding is worst. In fact, users can even filter data to identify school zones with elevated speeds specifically during school hours, ensuring the analysis focuses on the times children are present. This level of granular insight far surpasses traditional methods and ensures cameras address actual speeding problems, not just theoretical ones.
Traffic Volume and Congestion Trends
A location where 5 out of 10 cars speed might be less dangerous than one where 50 out of 100 cars speed. Geospatial AI pairs speed data with traffic volume data to gauge risk exposure. High volumes combined with high speeds indicate a greater likelihood of conflicts. Additionally, congestion patterns are considered – heavy congestion might actually slow vehicles (reducing speed risk but possibly increasing other concerns like jaywalking).
By understanding typical traffic flow around each school (e.g., peak vehicle counts in morning vs. afternoon, queue lengths, backups), the AI can distinguish between spots where speeding is an occasional issue versus those that are true trouble zones during busy periods. This ensures that cameras are placed not only where speeding occurs, but where it coincides with lots of vehicles (and thus more potential interactions with pedestrians).
Pedestrian Activity and Student Presence
A crucial factor is where and when students are walking. Geospatial AI incorporates data on pedestrian movement – whether from pedestrian counters, crossing guard reports, or proxy data like mobile location services – to estimate foot traffic in and around school zones. Not all parts of a school zone have the same pedestrian risk. There may be a popular crosswalk two blocks from the school that many students use (for example, heading to a nearby neighborhood or bus stop). The AI can highlight these areas by mapping common walking routes to and from each school.
Additionally, school schedules are integrated: the times of day when children are likely present (morning arrival, lunchtime for some campuses, afternoon dismissal, and any after-school activities). If data shows a lot of pedestrian activity crossing a particular road at 3:00 PM, the system flags that location especially if cars at that time are often speeding. By correlating pedestrian density with driver behavior, geospatial AI identifies enforcement locations where a camera could most directly protect pedestrians.
In short, it finds the intersections of high speed + high pedestrian presence = high danger, which are ideal spots for automated enforcement.
Crash History and “Near Miss” Incidents
Past collision data is a telling indicator of where serious problems have occurred. Urban SDK’s platform layers historical crash records and even citizen-reported near-miss incidents onto the map. If a certain crosswalk or road segment near a school has a history of accidents or frequent close calls, that’s a strong signal that enforcement or engineering fixes are needed there.
Urban SDK’s Collision Index provides a risk score for road segments based on historical crash data, helping highlight the most dangerous locations. Geospatial AI can use this to ensure speed cameras are considered at locations with a record of pedestrian injuries or frequent speeding-related collisions. Moreover, by analyzing patterns in the crash data (e.g., a cluster of rear-end crashes might indicate chronic sudden stopping, while a pattern of pedestrian injuries in late afternoon might correlate with after-school speeding), the system gives context to why a camera could be effective. It even looks at near misses – for example, if data from school crossing guards or traffic cameras indicates many instances of cars braking hard to avoid hitting pedestrians, that’s a location where a camera might prompt earlier slowing and prevent the near misses from becoming actual crashes.
Geospatial Risk Scoring and Mapping
The true power of Geospatial AI comes when it integrates all these data layers together. Urban SDK’s tools can overlay speed heatmaps, traffic volumes, pedestrian routes, and crash hotspots on a city map to visualize where risks converge. Through machine learning models, each potential camera location (say every block within a school zone) can be scored based on risk factors.
For instance, a segment might get a high score if it has above-limit speeds, high traffic during school times, many student pedestrians, and even a past incident. Another segment might score lower due to lower speeds or fewer pedestrians.
By scoring and ranking locations, the AI effectively prioritizes where a speed camera would likely have the greatest safety benefit. This objective, data-driven ranking helps transportation professionals make informed decisions. It can reveal, for example, that while every school zone has some speeding, these specific five locations city-wide stand out as the most critical for camera enforcement. Armed with that insight, agencies can allocate their camera installations to those spots first, confident that they are addressing the worst risks. This approach fulfills the FHWA’s Safe Routes to School recommendation to use “data collection and technology tools to target the most dangerous areas for children walking or biking”– geospatial AI makes that targeting possible at scale.
Adaptation to Real-Time Conditions
Another advantage is that our geospatial AI platform operates in real-time or near-real-time. They aren’t a one-and-done study; they continue to ingest new data. This means if conditions change – say a detour pushes traffic into a school zone one month, or seasonal events (like a county fair) spike traffic near a school – the AI can detect new speeding patterns and suggest adjustments. Cities can be proactive by temporarily rotating a mobile speed camera to a new hotspot identified by fresh data, for example.
The AI can also incorporate environmental conditions (weather, daylight, etc.) that might affect driver behavior. If data shows that on rainy days speeding increases on the road by the school (perhaps due to fewer pedestrians visible or drivers in a rush), authorities could decide to emphasize enforcement on those days. This level of responsiveness is far beyond traditional static placement strategies.
In summary, geospatial AI analyzes a rich tapestry of data – speeds, volumes, pedestrian movements, crashes, and schedules – to pinpoint optimal speed camera locations. By quantifying risk and likely impact, it takes the guesswork out of enforcement planning. Public leaders get a clear, evidence-based map of where a camera will do the most good, which is a game-changer for efficient and effective school zone safety programs.
Benefits of Strategic Speed Camera Placement
Strategically placing speed cameras with the help of geospatial AI yields numerous benefits for cities and their residents. By ensuring each camera is deployed where it can make the biggest difference, communities can expect to see improvements in driver behavior and safety outcomes. Here are some of the key benefits of this data-driven approach:
Reduced Speeding and Violations
The primary goal of speed cameras in school zones is to get drivers to slow down, and well-placed cameras achieve this. When locations are chosen based on actual speeding patterns, cameras serve as a strong deterrent where drivers habitually exceed limits.
The evidence is compelling – for example, New York City’s school zone speed camera program saw a 72% drop in speeding at camera locations on average. This means drivers dramatically reduced their speed in areas where cameras were watching. By targeting the most egregious speeding hotspots, cities can maximize this effect. Fewer drivers speeding translates to fewer violations issued over time (after the initial behavior change), which is actually a positive sign – it means drivers are complying with the law. The overall reduction in speeding directly lowers the probability of crashes and injuries.
In short, optimized placement “calms” traffic where it’s most dangerous, creating a safer driving environment throughout the school zone. And as drivers adjust their habits, the safety benefits can extend beyond the camera location (a phenomenon often called a halo effect, where drivers maintain lower speeds even after leaving the enforced zone).
Increased Driver Compliance and Safer Driving Culture
Strategic camera placement doesn’t just catch violators, it helps build a culture of compliance. When drivers know that the most risky school zones are actively enforced (and when they’ve perhaps received a warning or ticket from a camera), they become more conscious of their speed overall. An optimized network of cameras serves as an ever-present reminder to drivers that speeding in any school zone is unacceptable. Over time, this consistency leads to behavior change. Instead of treating a school zone speed limit as optional, motorists start to automatically slow down, whether a camera is visibly present or not. This was observed in many programs that driver behavior improves significantly near speed camera sites and stays improved even when enforcement isn’t obvious.
With geospatial analysis ensuring cameras are at the right spots, the public perceives the enforcement as fair and focused on safety (rather than a random “speed trap”). This can increase community support and voluntary compliance. The result is not just compliance to avoid tickets, but a genuine shift towards safer driving habits during school hours – exactly what we want around our children’s schools.
Enhanced Safety for Children and Pedestrians
Ultimately, the greatest benefit of optimized speed camera placement is the improvement in safety for students, parents, and school staff. By reducing speeding and calming traffic, the likelihood of a crash occurring in a school zone drops substantially. Locations that once posed high risk (a crosswalk where children frequently had close calls, or a stretch of road where a child pedestrian was struck in the past) become much safer under the watchful eye of a camera.
Studies have shown that motor vehicle collisions fall at locations near speed cameras, and injury rates drop as well. Slower speeds give drivers more reaction time and make it far more likely that if a collision does occur, it will be a minor one rather than a fatality. For children who must cross busy streets to get to school, this is life-saving. Parents gain peace of mind knowing that the city is actively protecting school routes.
In the bigger picture, safer school zones can encourage more kids to walk or bike to school, promoting healthy exercise, because parents feel more confident about traffic dangers being under control. The community benefits from fewer tragic incidents, and the streets around schools become welcoming environments rather than danger zones. Every child deserves a safe journey to and from school, and strategically placed speed cameras are a proven tool to deliver that safety.
Efficient Use of Resources
Another benefit worth noting is the efficiency gained by strategic placement. Speed enforcement cameras and their supporting infrastructure cost money to install and operate. Using geospatial AI to guide placement means cities can get the best “bang for the buck” from each camera. Rather than blindly installing devices and hoping for safety improvements, officials can be confident that each camera is going to a high-need location backed by data. This can also help in justifying the investment to stakeholders – when you can show that a camera is predicted to reduce crashes by X% at a specific site due to known issues, it builds a strong case. Additionally, by reducing collisions, cameras save money in the long run (less emergency response, medical, and property costs).
A Columbia University study even found speed cameras to be among the most cost-effective safety measures, suggesting that optimizing the number and placement of cameras can save millions in societal costs while protecting lives.
In practical terms, strategic deployment avoids wasting cameras in low-impact areas and focuses maintenance and operational efforts where they matter most. If a city has a limited number of cameras or enforcement units, geospatial analysis ensures those resources are deployed in the most impactful configuration.
Data-Backed Accountability and Community Trust
A side benefit of this approach is greater transparency and trust with the public. When a city uses a data-driven method to improve safety, it can share those insights with the community.
Urban SDK’s platform, for instance, allows creation of geospatial dashboards and maps that officials can use to explain why certain locations were chosen for camera enforcement. Showing parents and school boards the heatmaps of speeding or the crash history can build understanding that these cameras are truly for safety, not revenue. This kind of openness fosters community buy-in. It also allows for tracking results – because the same platform that identified the problem can measure the after-effects.
City leaders can report back, “Since installing cameras, average speeds in these school zones have dropped by 20% and violations by 72%. We’ve seen no crashes at these crossings in the past six months.” Such reporting is powerful for maintaining support and for making adjustments if needed. In essence, strategic placement guided by geospatial AI leads to evidence-based policymaking, where every enforcement decision is backed by analysis and can be evaluated for effectiveness.
By reaping these benefits – from slower cars and safer kids to more efficient enforcement – cities move closer to the vision of Vision Zero (zero traffic fatalities) within school zones. Geospatial AI gives communities the means to systematically protect their most vulnerable road users: children.
Urban SDK’s Role in Smarter School Zone Enforcement
Urban SDK helps cities:
- Map school zones dynamically with surrounding traffic and pedestrian data
- Analyze real-time speed patterns block by block
- Score road segments using crash history and behavioral trends
- Monitor and adjust camera zones over time based on updated data
Our platform integrates multiple data feeds into one intuitive dashboard, providing:
- Heatmaps of speeding during school hours
- Collision risk indexes for each road segment
- Pedestrian density overlays
- Before-and-after analysis of interventions
Next Steps for City Leaders
If you’re responsible for transportation safety in your community, here’s how to get started:
1. Audit Your School Zones
Gather all school zone locations, road classifications, current signage, and any existing enforcement data.
2. Connect Your Data Sources
Bring together speed, volume, crash, and pedestrian datasets. Don’t have them all? Urban SDK can help supplement with our traffic data library.
3. Use Urban SDK for Geospatial Analysis
Our platform makes it easy to visualize risks and prioritize zones based on multiple variables.
4. Build a Data-Backed Enforcement Plan
Start with the highest-risk locations. Present findings to stakeholders, school boards, and grant reviewers to gain buy-in.
5. Monitor, Report, and Adjust
Use real-time data to assess impact and modify placements over time. Transparency improves community support and long-term success.
By following these steps, public leaders can move confidently toward a safer future for school children. It starts with data and ends with on-the-ground action that saves lives. The technology and tools to do this are more accessible than ever – and the difference they can make is profound.
Urban SDK is ready to partner with forward-thinking communities to put these plans into motion. When we optimize school zone speed camera placement with geospatial AI, we turn information into impact – making streets safer for the youngest and most vulnerable among us.
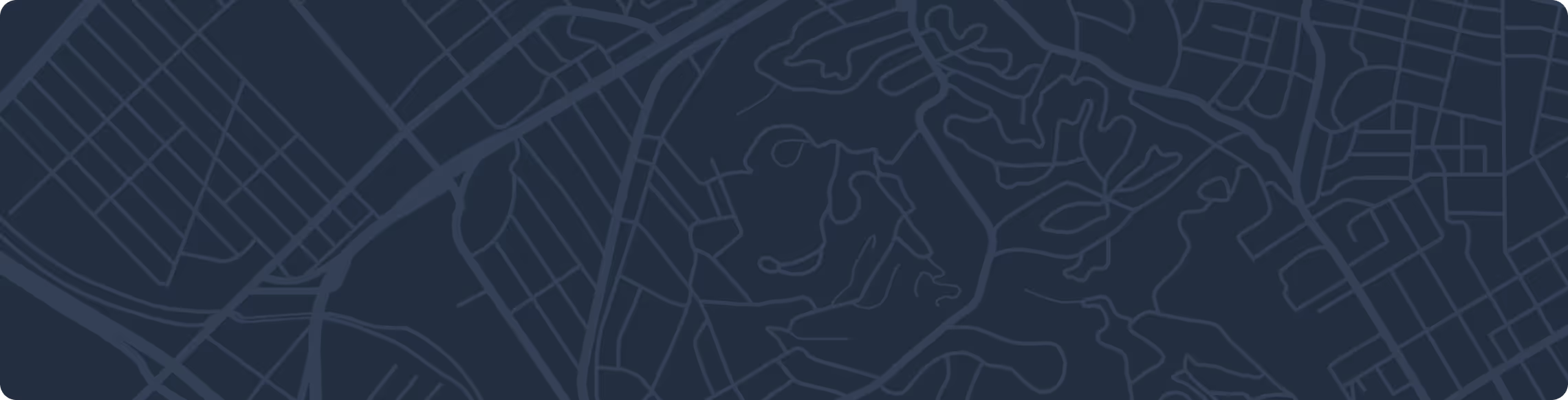
TRAFFIC ENFORCEMENT FEATURES
80% of citizen complaints
are a perception problem
Urban SDK provides precise hourly speed data to evaluate complaints and deploy resources efficiently for the greatest impact to public safety.
Urban SDK provides precise hourly speed data to evaluate complaints and deploy resources efficiently for the greatest impact to public safety.
Target Speeding
Identify hot spots, validate monthly speeding trends and monitor vulnerable areas like school zones.
Improve Safety
Crash and citations location information to compare speed trends month over month
Fast Response
Respond to citizen complaints sooner with address search and exportable reporting
Deploy Assets
Generate maps for traffic enforcement by time of day, location or division to deploy officers to known problem areas.
RESOURCES
Customer Success
See how public sector leaders succeed with Urban SDK.
WEBINAR
Identify speeding and proactively enforce issues
See just how quick and easy it is to identify speeding, address complaints, and deploy officers.
